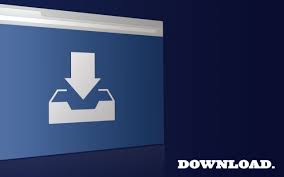
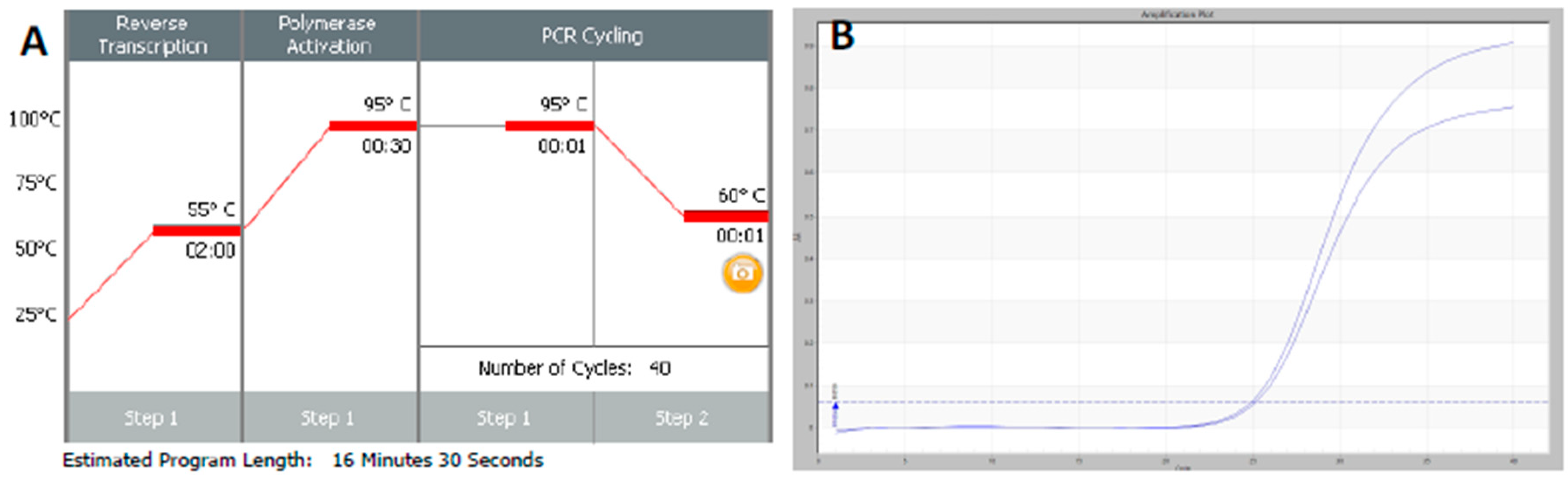
Performs best when applied to high throughput experiments? From this point, what existing dataĪnalysis methods can be ported to high throughput? What method High throughput qPCR requires analysis to beīoth fully automated and robust.
QPCR DATA ANALYSIS PROGRAMS MANUAL
Thousands of them raises concrete issues since manual intervention is However, going from one or a bunch of qPCR curves to As a consequence, they were mostly designed as semiautomated. Reaction could still be visually investigated and analysis manuallyĬurated. In the context of low throughput qPCR experiments, where each Understanding, a relative comparison of gene copy between samplesĬovers most applications. Usefulness is questionable in a high throughput context. While absolute quantification is, in theory, an interesting goal, its Order to extract meaningful information from qPCR curves. To date, numerous data analysis strategies have been proposed in In practice, whatever the throughput, qPCR producesĪmplification curves that need to be analyzed and quantified. That allow miniaturization of individual reactions at a nanoliter It followed the rise of microfluidic devices Years, the throughput of qPCR that could be performed in parallel has also significantly increased. Increased the precision of gene copy quantification. Monitoring the reaction in real time, thus allowing indirect access Reaction (qPCR) followed in the next decade. Development of real time or quantitative polymerasechain.

Technique with a broad set of applications in biological research and Generate billions of copies from a small DNA sample, is a widely used Polymerase chain reaction, designed in the mid 80’s in order to Keywords: DrugReceptor Interactions, Computational Approach While deep learning presents the advantage of not requiring any preprocessing steps, we nevertheless conclude that Cq, one of the oldest method, because of its simplicity, its robustness to dataset variability, and the fact that it doesn’t require a large training set, should be preferred over other approaches to analyze high throughput qPCR experiments. The advantages and disadvantages of these methods in this new context were discussed. Using four quantitative criteria, we then compared results obtained on high throughput experiments using five reference methods designed for low throughput qPCR data analysis (Cq, Cy0, logistic5p, LRE, LinReg) as well as deep learning. In order to perform such comparison on a common ground, we developed a preprocessing approach based on the design of a robust high throughput fitting to correct bias and baseline prior to further quantification of gene expression by individual methods. In this work, we aim to evaluate and compare available qPCR data analysis methods when ported to high throughput. In consequence, the community has not yet named a reference method to analyze HT-qPCR data. An extensive comparison of these methods in this new context has not yet been performed.
QPCR DATA ANALYSIS PROGRAMS FULL
Recently, modern platforms have largely increased the throughput of samples and a single HTqPCR experiment can produce up to ten thousand reaction curves, requiring full automation. For the past 25 years, real time quantitative PCR (qPCR) has been the method of choice to measure gene expression in biological research and diagnosis using semi-automated data analysis methods adapted to low throughput.
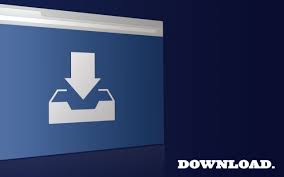